Every business today is racing to harness the immense power of data. Yet, managing fragmented and growing data ecosystems remains one of the most significant challenges for organizations worldwide. According to IDC, it is projected that by 2023, 95% of organizations will adopt private data KPIs in their digital strategies.(source)
To solve this challenge, two revolutionary frameworks have emerged: Data Fabric and Data Mesh. These approaches promise to transform how businesses access, govern, and derive value from their data, each with unique methodologies tailored to different needs.
In this post, we’ll understand the fundamental differences between Data Fabric and Data Mesh, analyze the business challenges they aim to solve, and help you determine which approach might drive better value for your organization. Understanding these frameworks is essential for making informed decisions in today’s complex data landscape.
Data Fabric vs. Data Mesh – Which Approach Drives Better Business Value
As organizations work towards unlocking the full potential of their data, the complexity of managing, integrating, and leveraging diverse datasets becomes a critical challenge. With data scattered across silos, systems, and geographies, businesses often struggle to extract meaningful insights at a scale. In this context, two emerging architectural paradigms, Data Fabric and Data Mesh, have gained prominence as potential solutions.
What is Data Fabric?
Modern organizations often face challenges with fragmented and siloed data sources, which hinder their ability to derive actionable insights and make informed decisions. Data Fabric offers a transformative solution to this problem. Acting as a technology-centric framework, it integrates disparate data sources using AI and automation, creating a unified and centralized ecosystem for data management.
This seamless integration ensures that organizations can access and utilize their data efficiently. To better understand how Data Fabric achieves this, let’s examine its key features and their impact on data ecosystems.
Key Features of Data Fabric
1. Centralized Integration: Consolidates fragmented systems into a unified data view, ensuring consistency and reliability.
2. Automation with AI/ML: Simplifies complex processes such as augmented data cataloging, metadata management, and governance.
3. Seamless Accessibility: Facilitates real-time data access across enterprise systems, improving operational efficiency.
By addressing common data management challenges, these features enable organizations to transform their fragmented data ecosystems into cohesive and efficient systems. However, while Data Fabric presents many advantages, it also has limitations that should be considered.
Advantages of Data Fabric
1. Enhanced Data Products: Integrates and automates data management platforms, ensuring delivery of consistent and reliable data products.
2. Faster Implementation: Builds on existing infrastructure, reducing the time required to realize value.
3. Compliance-Friendly: Maintains governance and regulatory standards, making it ideal for industries such as healthcare and finance.
These advantages highlight why Data Fabric is a game-changer for modern organizations. Yet, implementing such a framework is not without challenges.
Challenges with Data Fabric
1. Cost and Complexity: Requires substantial investment in AI-driven tools and resources.
2. Limited Cultural Impact: Focuses primarily on technology, often neglecting the need for organizational and cultural transformation.
Understanding these challenges helps organizations weigh the trade-offs and plan effectively for implementation.
Best Use Cases for Data Fabric
Data Fabric is particularly well-suited for industries that prioritize compliance and real-time data access, such as finance and healthcare. It is also a powerful tool for organizations seeking to enhance decision-making capabilities without the need for extensive cultural changes.
What is Data Mesh?
Organizations often struggle to balance centralized control with the agility needed to address diverse data demands. Data Mesh provides a solution by decentralizing data management responsibilities. Instead of relying on a single, centralized team, it empowers domain-specific teams or departments to take ownership of their data, treating it as independent products tailored to their unique needs.
This approach fosters collaboration, accountability, and usability, enabling organizations to unlock the full potential of their data assets. To fully appreciate the impact of Data Mesh, let’s explore its key features, advantages, challenges, and ideal use cases.
Key Features of Data Mesh
1. Decentralized Ownership: Empowers domain-specific teams to handle their own data management, fostering autonomy.
2. Data as a Product: Ensures high-quality, discoverable, and user-friendly datasets that meet organizational needs.
3. Collaboration-Oriented: Encourages a cultural shift towards accountability and domain expertise, driving better outcomes.
These features make Data Mesh a powerful framework for organizations seeking to improve data usability and adaptability.
Advantages of Data Mesh
1. Agility in Data Product Development: Allows teams to create and adapt data products rapidly to meet evolving needs.
2. Scalability: Distributes data management responsibilities, reducing bottlenecks and enabling faster innovation.
3. Innovation Driven: Promotes collaboration and domain-specific insights, driving transformative ideas and solutions.
The advantages of Data Mesh help organizations become more responsive and innovative in addressing data challenges.
Challenges with Data Mesh
1. Organizational Transformation: Requires a significant cultural shift, including data literacy across teams and buy-in from stakeholders.
2. Governance Complexity: Balancing autonomy with compliance and security can pose challenges, especially in regulated industries.
Understanding these challenges is crucial for successful implementation and maximizing the benefits of Data Mesh.
Best Use Cases for Data Mesh
Data Mesh is best suited for large, decentralized organizations with diverse and complex data needs. It excels in environments that prioritize agility, collaboration, and cultural transformation, making it ideal for enterprises aiming to modernize their data strategies.
By decentralizing data management and fostering collaboration, Data Mesh helps organizations adapt to changing demands while empowering teams to take ownership of their data-driven initiatives.
Comparing Data Fabric and Data Mesh
As organizations navigate the complexities of modern data management, both Data Fabric and Data Mesh have emerged as prominent frameworks, each addressing unique challenges. While both aim to improve data accessibility, usability, and integration, they differ significantly in their approach and focus. Understanding the distinctions and overlaps between these two paradigms is essential for organizations seeking to select the right framework based on their operational needs, scalability goals, and cultural dynamics.
By comparing their core principles, advantages, and ideal use cases, businesses can make informed decisions about which approach aligns best with their data strategy. Let’s delve into the key aspects of Data Fabric and Data Mesh to better understand their similarities, differences, and relevance.
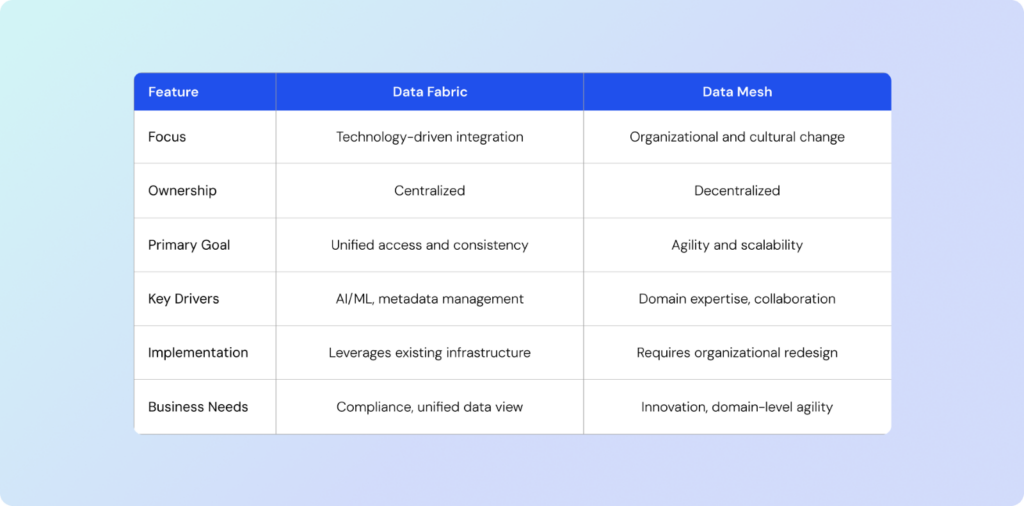
Choosing the Right Approach for your Business
The stakes are high. Choosing the right approach affects not just how data is managed but how quickly and effectively it can deliver value. Organizations must weigh factors like scalability, governance, cost, and cultural readiness when deciding which paradigm to embrace. Missteps can result in fragmented efforts, spiraling costs, and missed opportunities to leverage data as a competitive advantage.
Despite their promise, these approaches are not without their own challenges. For instance, businesses adopting Data Fabric often face difficulties with its heavy reliance on centralized integration and automation, which can lead to bottlenecks and limited flexibility in highly dynamic environments. On the other hand, the Data Mesh philosophy, with its decentralized, domain-oriented model, can create governance and interoperability challenges, especially for organizations without strong data ownership cultures.
When to choose Data Fabric
Data Fabric is ideal for organizations seeking to centralize their fragmented data systems. It offers a structured and consistent way to manage data, making it easily accessible and reliable. This framework is particularly beneficial for businesses that prioritize compliance and governance, as it provides robust oversight of data processes. Furthermore, if your organization has the resources to invest in AI-driven technologies, Data Fabric ensures faster implementation and real-time insights.
Here are key scenarios when Data Fabric is a good fit:
- You need a centralized, real-time data view
- Compliance and governance are high priorities
- You have resources to invest in AI-driven technologies
When to choose Data Mesh
Data Mesh is a perfect fit for businesses that value flexibility and innovation in their data management approach. It empowers domain teams to take ownership of their data, fostering accountability and tailored solutions. If your organization is ready to embrace cultural and operational changes, Data Mesh enables agility and decentralization. Additionally, this framework is ideal for large organizations with diverse data needs, where collaboration and domain-level expertise are critical.
Below are situations where Data Mesh excels:
- Your organization values decentralization and agility
- Teams are ready to embrace cultural and operational changes
- Innovation and domain-specific ownership are key priorities
A Hybrid Approach
For many businesses, a hybrid model combining Data Fabric and Data Mesh can offer the best results. For example:
- Use Data Fabric to manage centralized integration and governance
- Apply Data Mesh principles to enable domain-level agility and innovation
This approach ensures scalability and efficiency while fostering collaboration and accountability.
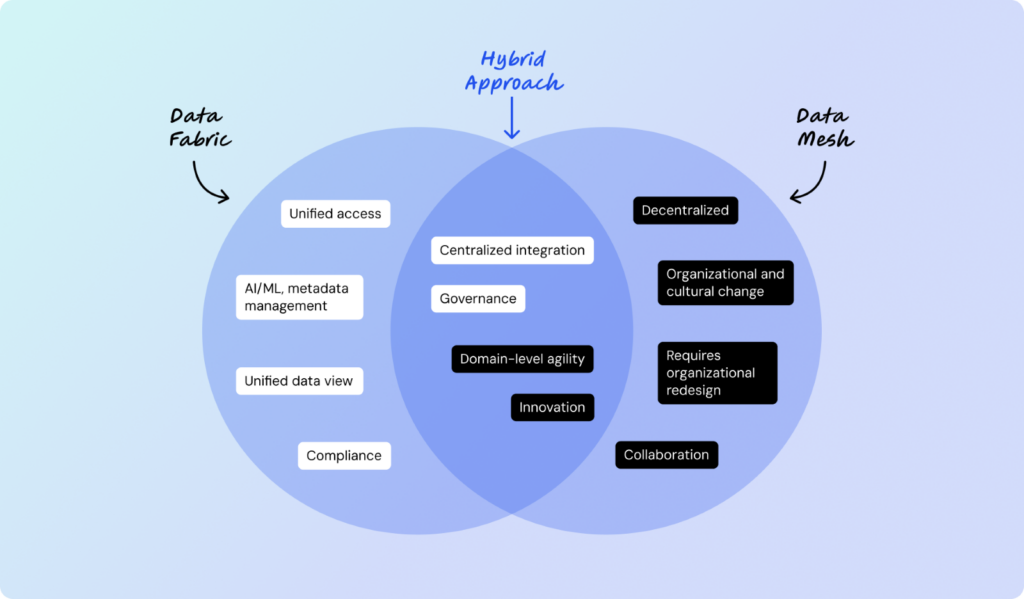
The Role of Arivonix in Data Management
Arivonix bridges the gap between these frameworks, providing strong data management platforms tailored to organizational needs. Here’s how:
- Data Cleaning and Preparation: Automates processes to ensure high-quality data.
- Data Modeling and Analysis: Delivers insights with advanced AI-driven tools
- Data Product Development: Empowers teams to create scalable, reliable data products.
- Seamless Integration: Connects with existing infrastructures to simplify deployment.
With Arivonix, businesses can adopt scalable, reliable, and efficient data strategies that combine the strengths of Data Fabric and Data Mesh.