Businesses that rely only on historical data are always a step behind. Generative AI in data analytics is changing how businesses make use of their data by identifying patterns and predicting outcomes for proactive, data-driven decisions. Instead of reacting to past trends, businesses are now anticipating shifts and adjusting strategies before they happen.
With this predictive analytics capability at their fingertips, let’s dive into how businesses are applying generative AI to refine their decision making AI models and achieve tangible results.
Understanding Decision-Making AI
Businesses today generate more data than ever, but data alone doesn’t drive success – how it’s interpreted and acted upon does. Traditional decision making relies on human intuition and retrospective analysis, which are often slow, inconsistent, and limited by cognitive biases. In fast-moving industries, this approach is no longer sufficient.
Decision making AI exists because businesses need to process vast amounts of information in real-time, identify patterns beyond human capability, and automate strategic choices with higher accuracy.
Whether it’s about mitigating financial risk, optimizing supply chains, or personalizing customer experiences, AI helps companies make smarter, faster, and more scalable decisions.
The demand for Decision Making AI Models is growing. Let’s explore some key benefits that these data backed AI models bring to the table.
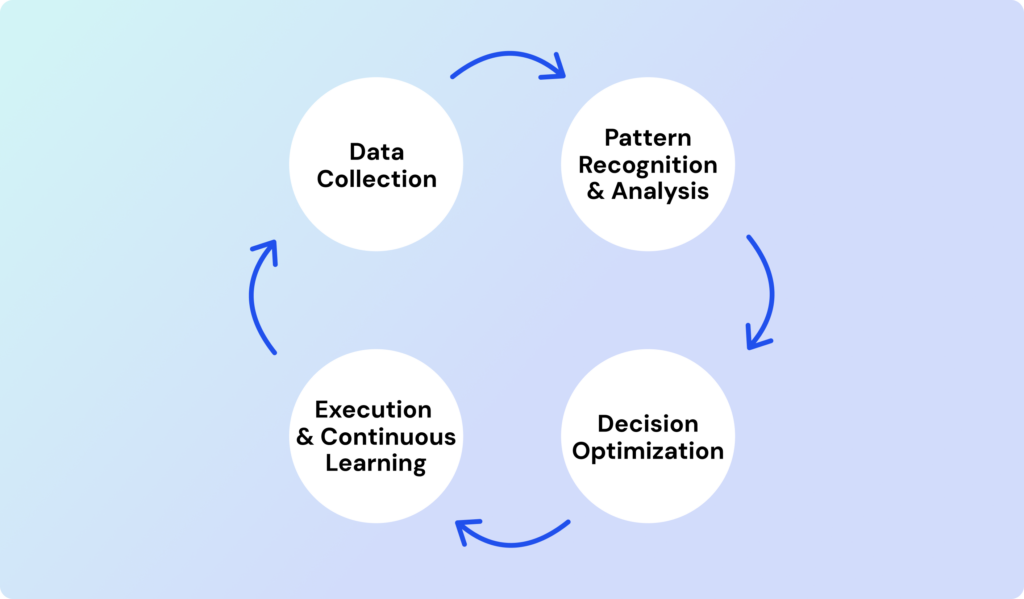
Key Benefits of Decision Making AI Models
Decision making ai enhances business operations by:
- Speed and Responsiveness: AI analyzes data rapidly, enabling quick adjustments to strategies that capitalize on market opportunities. For example, financial trading platforms use AI to suggest split-second trading decisions.
- Scalability: AI systems manage increasing data volumes without the need for more human resources, supporting complex, global operations. For example, e-commerce platforms use AI to dynamically scale customer support chatbots during high-traffic periods, reducing overhead costs and more.
- Consistency: AI ensures precision and uniformity in tasks requiring high reliability, such as quality control and compliance. For instance, manufacturing companies use AI-powered inspection systems to detect defects in products with near-perfect accuracy, reducing downtime and ensuring quality standards.
- Predictive Capabilities: By forecasting trends, AI prepares businesses for future challenges and opportunities, ensuring a competitive edge. For example, In healthcare, AI is used to analyze patient data and predict disease outbreaks, allowing for proactive intervention and resource planning.
- Cost Efficiency: Automation reduces labor costs and minimizes errors, and enables data monetization. For example, logistics companies leverage AI to optimize delivery routes in real time, reducing fuel consumption and operational costs.
These advantages position businesses to not only understand and react to their current environment but also to plan and execute strategies that anticipate future conditions.
While AI driven decision making improves the way businesses respond to challenges, its true potential is unlocked when it operates within a fully integrated data ecosystem. AI-powered unified data applications take this a step further by centralizing insights, automating workflows, and driving business profitability at scale.
How AI-Powered Data Apps Drive Business Profitability
Modern enterprises generate massive amounts of data across multiple platforms – CRM systems, financial software, IoT devices, and customer interactions. However, fragmented data limits efficiency, slows decision-making, and reduces profitability.
AI-powered unified data applications solve this by centralizing and processing data in real-time, transforming raw information into high-impact business intelligence.
Here’s how:
- Eliminating Data Silos: AI-driven applications consolidate structured and unstructured data from multiple sources into a unified framework, creating a single source of truth for all business functions. This prevents operational misalignment, enhances collaboration, and ensures consistent data governance.
For example, global enterprises integrate AI-powered data platforms to unify customer interactions across regions. This enables hyper-personalized marketing and real-time customer insights, driving higher engagement and revenue growth.
- Real-Time Decision Intelligence: Unlike traditional analytics tools that rely on periodic data refreshes, AI-powered systems continuously ingest and analyze live data streams. Businesses can instantly respond to market fluctuations, supply chain disruptions, and shifting consumer behavior—reducing risk and unlocking revenue opportunities.
For instance, investment firms leverage AI to assess market volatility in real-time, adjusting portfolios dynamically to optimize returns while mitigating risk exposure.
- Automating Data-Driven Actions: AI doesn’t just analyze data—it automates complex decision workflows. From predictive maintenance in manufacturing to automated fraud detection in banking, AI enables businesses to reduce operational bottlenecks, improve precision, and increase profitability.
For example, industrial manufacturers use AI-driven predictive maintenance systems that forecast equipment failures before they occur, reducing unplanned downtime and saving millions in lost production costs.
- Optimized Resource Allocation: AI ensures that businesses allocate capital, manpower, and operational resources with maximum efficiency. AI-powered forecasting eliminates inefficiencies in marketing, supply chain, and logistics.
For example, logistics firms use AI to optimize delivery routes based on real-time traffic, weather conditions, and cost modeling—reducing fuel expenses while improving last-mile delivery efficiency.
- Enhanced Security and Compliance: AI-powered anomaly detection and automated compliance monitoring help businesses prevent financial losses and regulatory violations. By continuously scanning transactional patterns, AI can detect threats before they escalate.
For instance, financial institutions deploy AI-driven fraud detection systems that identify and flag high-risk transactions within milliseconds, preventing revenue losses and safeguarding compliance with regulatory frameworks.
By transforming scattered data into a highly structured, intelligent ecosystem, AI-powered unified data applications drive smarter decision-making, reduce costs, and create new revenue opportunities.
But AI isn’t stopping here. As businesses continue adopting AI driven decision making, the technology itself is evolving – becoming more adaptive, autonomous, and capable of making high-impact decisions with minimal human intervention.
Want to learn more about how AI is transforming data monetization?
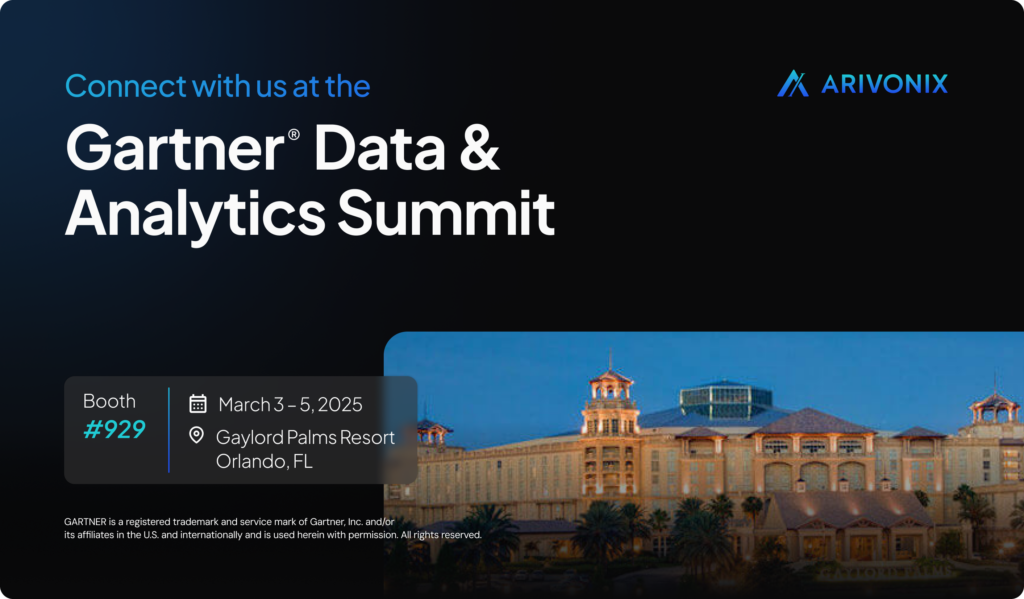
The Future of Decision Making AI
AI driven decision making is evolving rapidly, shifting from simple automation to complex, adaptive intelligence that continuously refines itself. The future of AI driven decision making is defined by three key advancements:
- Self-Learning AI Models: Traditional AI relies on pre-set rules and training data. Next-generation AI will self-learn in real time, adjusting its decision making logic based on live data. This means businesses won’t just automate decisions – they’ll have AI systems that improve and evolve without human intervention.
- Context-Aware Decision Systems: AI will move beyond pattern recognition to true context awareness. Instead of making decisions solely based on numbers, AI will factor in external influences such as economic conditions, regulatory changes, and shifting consumer behavior. This will make AI-powered decisions more dynamic, reducing risk and improving long-term strategic planning.
- Autonomous Decision Execution: AI will not just suggest recommendations—it will act on them. Whether adjusting supply chain logistics, modifying pricing strategies, or allocating resources in real-time, AI will execute complex decisions with minimal human oversight. This will allow companies to scale operations efficiently while reducing manual intervention.
As businesses continue to embrace decision making AI, decisions will shift from reactive to proactive. Organizations that invest in these advancements early will not only improve efficiency but also gain a competitive edge in rapidly changing markets.
Conclusion
Businesses that still rely on fragmented data and manual workflows will struggle to keep up as industries shift toward automation and real-time intelligence.
The ability to unify data, predict trends, and automate decisions at scale isn’t a luxury; it’s the new standard. Companies that invest in AI-powered search intelligence will gain a long-term competitive edge – operating faster, smarter, and with greater precision.
As decision making AI continues to evolve, the question isn’t whether businesses should adopt it, but how quickly they can integrate it to stay ahead.